Recent developments at the intersection of deep learning and toxicological image analysis herald a new era of efficiency and accuracy in high-throughput chemical screening. At Sciome, we are working with our clients to exploit the transformative potential of deep learning to solve important problems in this domain. Below we highlight a few recent projects which showcase Sciome’s commitment to addressing the challenges inherent in the analysis of large volumes of image data to provide efficient, standardized, and objective solutions. The results of this work serve not only to automate and enhance the accuracy of image-based assessments but also pave the way for innovative approaches to experimental design and benchmark dose calculations.
Sciome’s foray into deep learning for toxicological image analysis is indicative of a larger paradigm shift happening in scientific research. As the scientific community looks towards more advanced and streamlined methodologies, Sciome stands at the forefront, helping to steer the course towards a future where deep learning transforms toxicological assessments and accelerates the pace of scientific discovery.
Automated Classification of Cell Morphology Changes
Sciome has developed a platform that addresses the inherent challenge of using advanced imaging platforms to perform high-throughput chemical screening. Implementing this approach, would normally require human experts to analyze thousands of images of cell cultures treated with various chemicals, making this approach a costly and time-consuming procedure. By employing deep learning Convolutional Neural Networks (CNNs), our platform automates the classification of digital assay images, alleviating the subjectivity and time constraints of manual processes. With more than 98% accuracy, the resulting binary classifier can effectively distinguish between healthy and altered cell states for important model systems including differentiated and proliferated HepaRG cells. The multi-class classifier further refines this assessment, assigning granular labels with over 95% accuracy.
This work has been recently published in a study that meticulously explores various CNN architectures, showcasing Sciome’s commitment to optimizing performance [1-4]. The utilization of Class Activation Maps (CAM) adds a layer of transparency, allowing for a deeper understanding of the neural network’s inner workings and leading to additional algorithmic refinements. The results underscore the platform’s capability to deliver highly accurate and reproducible cytotoxicity assessments across diverse cell types, setting a benchmark for automated image classification in chemical screening.
Enhancing Zebrafish Embryo Developmental Toxicity Bioassay
Zebrafish are widely used as a model organism in biomedical research, especially for high-throughput screening of drugs and genes. Interest in zebrafish as a model organism has been actively growing at an annual rate of 13% over the past 15 years, as measured using peer-reviewed publications. They are routinely used to study various biological processes, human diseases and have contributed to many medical and scientific breakthroughs, such as gene therapy, organ regeneration, and drug discovery. We are working with our clients to explore the challenges of visually assessing larval morphological changes in zebrafish embryos—a resource intensive process prone to subjectivity. Here, deep learning again comes to the forefront as a solution. We have developed a customized multi-view convolutional neural network (MVCNN) which can take advantage of the distinct dorsal and lateral views of each embryo to achieve higher accuracy than conventional single view CNN models. As a result, we are able to automatically classify several common developmental malformations with high accuracy. This not only expedites the assessment process but also ensures a more objective and standardized output. As an added dimension, Sciome is developing a segmentation model to automatically identify and measure larval organ structures, promising a holistic and comprehensive evaluation of toxicological effects in zebrafish. Zebrafish image analysis represents a cutting-edge New Approach Methodology (NAM) in scientific research. The models developed epitomize Sciome’s commitment to leveraging deep learning for the advancement of programmatic decisions in chemical evaluation, offering a paradigm shift in efficiency and reliability. Results of this ongoing work are highlighted in several recent publications [5-6].
Accelerating Benchmark Dose Calculation through Deep Learning
Sciome is also making important efforts to address the resource-intensive nature of calculating benchmark dose values, traditionally reliant on conventional methods and apical endpoints or transcriptomic datasets. We have introduced a method that employs deep learning to classify assay images based on cell health, ultimately achieving over 98% accuracy. After an appropriate statistical transformation, the resulting model predictions can be used as in silico endpoints for the purpose of Benchmark Dose (BMD) modeling. Remarkably, the correlation between image-derived BMD values and those calculated using transcriptomic datasets surpasses 90%, suggesting the viability of high-throughput imaging as a faster and more efficient alternative.
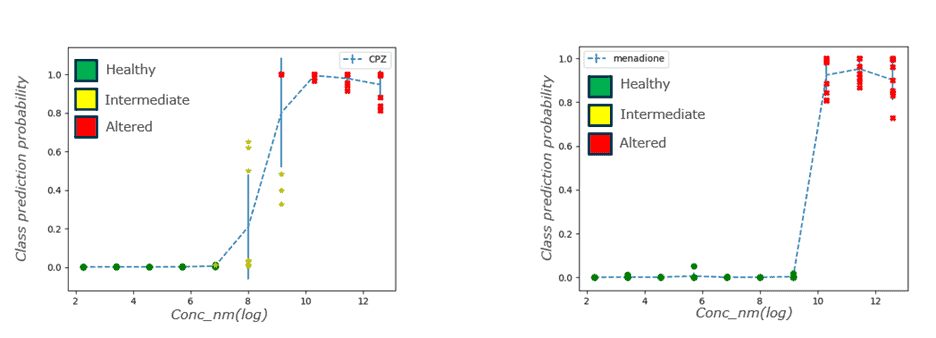
Pathway enrichment analysis of correlated transcriptomic responses further sheds light on potential applications, emphasizing the broad implications of Sciome’s deep learning approach in guiding experimental design. Results of this recent work are highlighted in a recent publication [7].
Publications and Presentations
Tandon A, Howard B, Ramaiahgari S, Maharana A, Ferguson S, Shah R, Merrick A (2022). “Deep Learning Image Analysis of High-Throughput Toxicology Assay Images”. SLAS Discovery 1:9; 27-38.
Tandon, A., Howard, B., Ramaiahgari, S., Maharana, A., Ferguson, S., Shah, R., Merrick, A. (2022). “Deep Learning Image Analysis of High-Throughput Toxicology Assay Images”. Poster at Society of Toxicology 2022 San Diego, CA. Selected as 2022 CTS Top 10 poster.
Tandon, A., Maharana, A., Howard, B., Ramaiahgari, S., Dunlap, P., Rice, J., Merrick, A., DeVito, M., Ferguson, S., Shah, R. (2020). “Towards Automation of High-Throughput Toxicology Assay Image Analysis Using Deep Learning”. Oral presentation at ASCCT 9th Annual Meeting.
Maharana, A., Howard, B.E., Tandon, A., Ramaiahgari, S., Dunlap, P., Rice, J., Merrick, A., Devito, M., Ferguson, S., Shah, R. (2019). “Deep Learning Image Analysis of High-Throughput Toxicology Assay Images”. Poster at Society of Toxicology 2019 Baltimore, MD
Tandon A, Howard B, Green A, Merrick A, Shah R, Shockley K, Cunny H, Ryan K, Hsieh H. Artificial Intelligence (AI)-Driven Morphological Assessment of Zebrafish Embryos for Developmental Toxicity Chemical Screening. Prep. (2024).
Tandon A, Howard B, Green A, Merrick A, Shah R, Shockley K, Cunny H, Ryan K, Hsieh H. Artificial intelligence (AI)-Driven Morphological Assessment of Zebrafish Embryo for Developmental Toxicity Chemical Screening. Society of Toxicology’s 63rd Annual Meeting and ToxExpo. Accepted Poster. (2024)
Tandon, A., Howard, B., Mav, D., Balik-Meisner, M., Ramaiahgari, S., Ferguson, S., Shah, R., Merrick, A. (2023). ” Deriving Benchmark Dose from the Deep Learning Prediction Scores of High-Throughput Toxicology Images”. Podium presentation at Society of Toxicology 2023 Nashville, TN.