During screening, as articles are included or excluded, an underlying statistical model automatically computes which of the remaining unscreened documents are most likely to be relevant. This “Active Learning” model is continuously updated during screening, improving its performance with each article reviewed. Meanwhile, a separate statistical model estimates the number of relevant articles remaining in the unscreened document list. The combination of the two models allows users to screen relevant documents sooner and provides them with accurate feedback about their progress. As a result, the majority of relevant articles can be discovered after reviewing only a fraction of the total number of abstracts, which can result in significant time and cost savings, particularly for large projects.
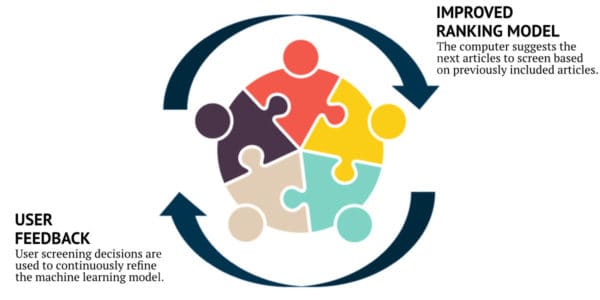