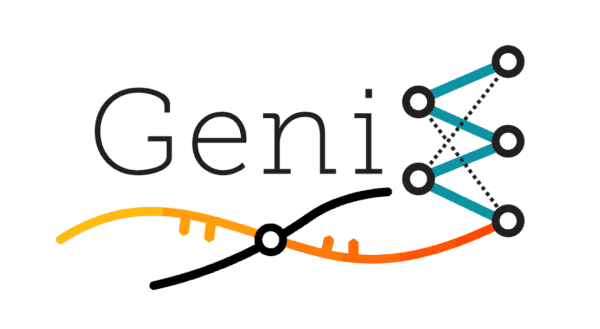
Gene Expression Analysis with GeniE
Comprehensive analysis of TempO-Seq data using novel, validated and robust bioinformatics methods. Upload raw Tempo-Seq data and instantaneously obtain in-depth analysis including cluster plots, principal component plots, differentially expressed gene list, and pathway analysis. In addition, GeniE offers extrapolated (predicted) full transcriptome from TempO-Seq measurements performed on a much smaller set of genes, such as the S1500+ platform.
- Pre- and post-extrapolation array of normalized expression data along with gene annotations
- Pre- and post-extrapolation QC outputs
- Density plots
- Cluster plots for user-annotated samples
- Scatter plots for user-annotated samples
- Principle Component Analysis (PCA) tabular output, PCA plots, and box and whisker plots for user-annotated samples
- Differentially expressed genes (DEGs) for all user-defined contrasts
- Differentially enriched pathways (DEPs) for all user-defined contrasts
Sentinel Genes
The typical transcriptome is comprised of tens of thousands of genes and expression of genes is highly correlated under varied conditions (Kohn et al. 2012; Judson et al. 2012; Ping et al. 2015; Li et al. 2014). Given this interdependence in expression, knowledge of the expression levels of a carefully chosen collection of sentinel genes provides sufficient information to accurately extrapolate estimates of the expression of the remaining genes. Therefore, a set of sentinel genes, coupled with a machine learning procedure, serve as a close proxy for whole-transcriptome expression, enabling accurate estimation of expression across the entire transcriptome. This enables measurement of more samples while spending less per sequenced sample. More information and publications can be found here (10.1371/journal.pone.0191105).
How is GeniE helpful?
TempO-Seq has emerged as an alternative to traditional RNA-Seq for high-throughput transcriptomics (Yeakley et al. 2017). A TempO-Seq experiment differs from standard RNA-Seq data sets in both the complexity of the sequence space (TempO-Seq uses only 50bp probe sequences) and the number of features for which read counts need to be computed and normalized. The effects of these design considerations on alignment and normalization algorithms and downstream analysis have been assessed, and GeniE applies optimal methods for the analysis of TempO-Seq data.
Additionally, extrapolation from a smaller number of (sentinel) genes to the whole transcriptome by GeniE expands your data, enabling various whole transcriptome-based analysis tasks, such as detection of more differentially expressed genes and pathways, robust dose response or time series analyses, and myriad of scientific investigations relying on measurement of the full transcriptome. This enables researchers to spend less on sequencing while maximizing their data analysis capabilities.
How GeniE works
GeniE provides extrapolation, differential expression testing, and pathway analyses with visual and tabular outputs. The extrapolation models in GeniE are based on the deeply interdependent nature of gene expression in the transcriptome. In other words, GeniE’s AI engine is trained on a very large amount of transcriptomics data and this enables GeniE to use expression signal from a small fraction (about 5-15%) of the representative genes, and accurately predict gene expression for the rest. Additionally, GeniE will perform differentially expressed gene (DEG) and differentially enriched pathway (DEP) analysis using user-provided contrasts to define pairwise comparisons of groups of samples.
Project Progress
GeniE’s project management page makes it easy to view your projects’ progress as your analyses are underway; and to retrieve results of completed projects.
No Need to Sit Around Waiting!
Immediate emails will be sent to your email account after submission and at job completion.
Afterward you can log back in to view your completed jobs and download results including extrapolated full transcriptome data.
Publications and Presentations
- Mav D, Everett LE, Balik-Meisner MR, Phadke D, Shah M, Ross A, Phillips J, Shah RR (2019). “Whole Transcriptome Extrapolation and Mechanism of Action Analysis using GENIE Pipeline” Society of Toxicology Meeting. Baltimore, Maryland, March 10-14, 2019.
- Mav D, Shah RR, Howard BE, Auerbach SS, Bushel PR, Collins JB, Gerhold DL, Judson RS, Karmaus AL, Maull EA, Mendrick DL, Merrick BA, Sipes NS, Svoboda D, Paules RS (2018). “A hybrid gene selection approach to create the S1500+ targeted gene sets for use in high-throughput transcriptomics.” PLoS One; 13-2, e0191105
- Mav D, Tandon A, Phillips J, Phadke D, Howard BE, Shah RR (2018). “Performance Evaluation of High-Throughput Transcriptome Extrapolation Techniques” Society of Toxicology Meeting. San Antonio, Texas, March 11-15, 2018.
- Mav D, Tandon A, Phillips J, Phadke D, Howard BE, Shah RR (2017). “Performance Evaluation of High-Throughput Transcriptome Extrapolation Techniques” Genetics and Environmental Mutagenesis Society of North Carolina Meeting. Durham, NC, Nov. 7, 2017.
- Shah R, Mav D, Judson R, Auerbach SS, Svoboda DL, Howard BE, Karmaus A, Gerhold D, Sipes NS, Collins J, Maull EA, Bushel PR, Merrick BA, Mendrick DL, Thomas RS, Paules RS (2016). “High-throughput Transcriptome Extrapolation via Sentinel Genes” Society of Toxicology Meeting. New Orleans, Louisiana, March 13-17, 2016.
- Shah R, Mav D, Judson R, Auerbach SS, Svoboda DL, Karmaus A, Gerhold D, Sipes NS, Collins J, Maull EA, Bushel PR, Merrick BA, Mendrick DL, Thomas RS, Paules RS (2015). “Gene Selection for Tox21 High-Throughput Transcriptomics” Society of Toxicology Meeting. San Diego, California, March 22-26, 2015.
References
Judson R. S., Mortensen H. M., Shah I., Knudsen T. B., and Elloumi F., “Using pathway modules as tar- gets for assay development in xenobiotic screening.,” Mol. Biosyst., vol. 8, no. 2, pp. 531–42, Feb. 2012.
Kohn K. W., Zeeberg B. R., Reinhold W. C., Sunshine M., Luna A., and Pommier Y., “Gene Expression Profiles of the NCI-60 Human Tumor Cell Lines Define Molecular Interaction Networks Governing Cell Migration Processes,” PLoS One, vol. 7, no. 5, p. e35716, May 2012.
Li J. et al., “Integrated systems analysis reveals a molecular network underlying autism spectrum disorders.,” Mol. Syst. Biol., vol. 10, no. 12, p. 774, Dec. 2014.
Ping Y. et al., “Identifying core gene modules in glioblastoma based on multilayer factor-mediated dysfunctional regulatory networks through integrating multi-dimensional genomic data,” Nucleic Acids Res., vol. 43, no. 4, pp. 1997–2007, Feb. 2015.
Yeakley J. M. et al., “A trichostatin A expression signature identified by TempO-seq targeted whole transcriptome profiling,” PLoS One, vol. 12, no. 5, p. e0178302, May 2017.