The American Society for Cellular and Computational Toxicology (ASCCT) 9th Annual Meeting, being held virtually October 20-22, aims to eliminate reliance on mammalian tests to make regulatory decisions. As part of this meeting, Arpit Tandon and Ruchir Shah will present recent work focused towards automation of high-throughput toxicology assay image analysis using deep learning. Automated approaches to perform high throughput toxicology often employ microscopy to capture photomicrographs from multi-well cell culture plates generating thousands of images that require time-consuming human analysis. To automate this subjective and time-consuming manual process, Sciome and NIEHS researchers have developed a method that uses deep learning to automatically classify digital assay images. By training a convolutional neural network (CNN) to perform binary and multi-class classification, testing and fine-turning various CNN architectures, and employing Class Activation Maps (CAM), deep-learning based image classification of cell toxicity can yield highly accurate results that are automated and reproducible.
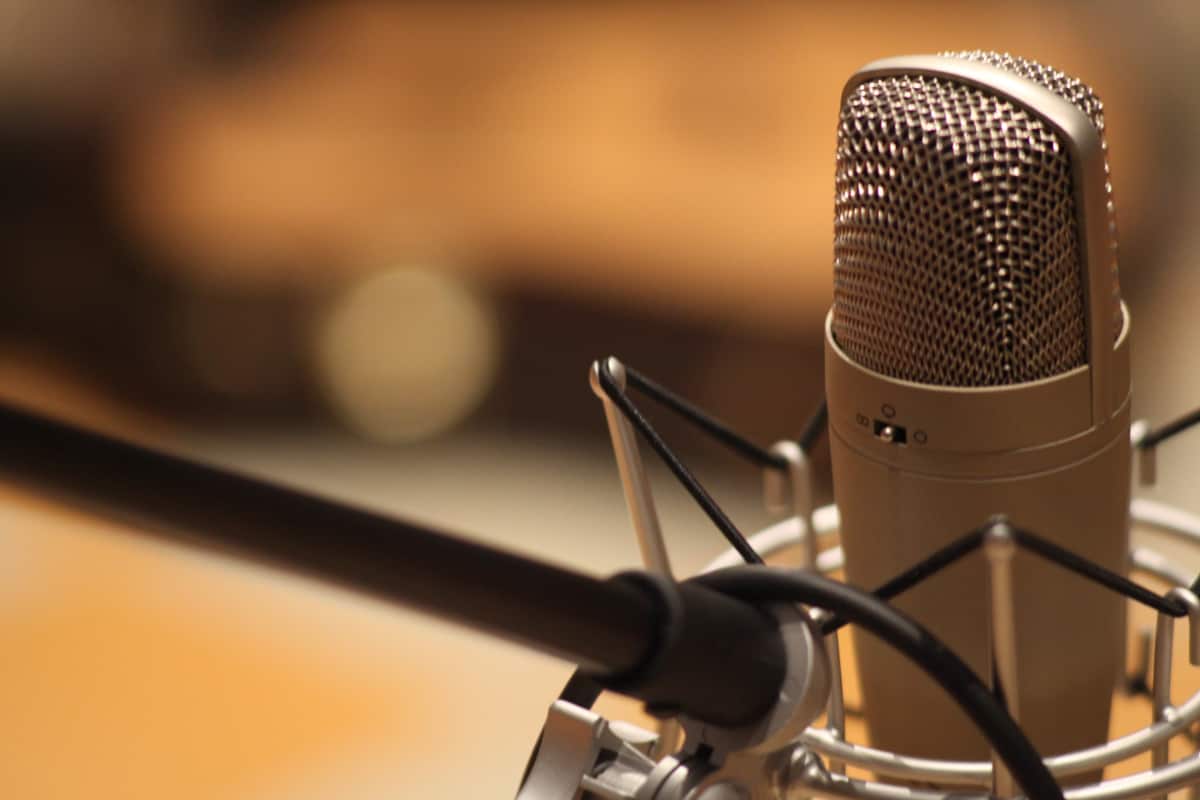