Saagar – A New, Extensible Collection of Molecular Substructures for Cheminformatics
Saagar is a compilation of molecular substructures for cheminformatics applications, including read-across assessments and interpretable Quantitative Structure-Property Relationship (QSPR) models. Saagar structural features have been systematically gathered by studying and reviewing open source literature that highlights relationships between sub-structural moieties and a variety of physico-chemical; absorption, distribution, metabolism and excretion (ADME); and toxicological properties of molecules.
The current edition of Saagar, SGR-0120, contains 834 chemistry-aware and chemically viable functional groups and moieties that perform better than commonly used fingerprints in chemically based read-across assessment [1] and in building interpretable QSAR models [2].
Saagar
Saagar is a compilation of molecular substructures for cheminformatics applications, including read-across decisions and interpretable Quantitative Structure-Property Relationship (QSPR) models. In contrast to using automatic fragmentation or atom- or bond-tracking methods to enumerate substructures in a molecule, Saagar uses pre-defined, chemistry aware and chemically viable diverse functional groups, moieties and scaffolds. Most of the Saagar features have been systematically retrieved from literature highlighting their relationship with physico-chemical; absorption, distribution, metabolism and excretion (ADME); and toxicological properties of molecules. In general, Saagar features capture salient structural attributes of a molecule, namely the hierarchical macro structural class (inorganic, aliphatic – acyclic or alicyclic, aromatic – fused or unfused carbo-aromatic and hetero-aromatic, and organometallic), elemental makeup, ring size, ring substituents and their positions, the extent of topological separation of certain hetero atoms, and scaffolds of endogenous biochemicals and common medicinal and industrial chemicals.
Structure Coverage by Saagar
Because the universe of chemicals is unbounded, it is not possible to devise substructures and moieties that would completely cover all structural aspects. However, large benchmark compound sets (Tox21, DrugBank, ChEMBL) were used to ascertain sufficient coverage by Saagar features. A few examples of the coverage provided by some Saagar features are shown below.
Active Enrichment by Saagar
Finding a data-rich compound structurally “similar” to a data-poor compound is fundamental to successful read-across prediction. Efficiency of Saagar features in identifying molecules similar in structure and activity to a given target has been tested using three benchmark groups of compounds covering >140,000 compounds spanning 78 screens. Measured in terms of enrichment fold (EF), the Saagar features returned better efficiency than that obtained with four well-known fingerprints, namely ECFP4 (1024 bits), MACCS (166 bits), PubChem (881 bits), and ToxPrint (729 bits) at 1%, 2%, 5%, and 10% false discovery rates (FDRs) [1].
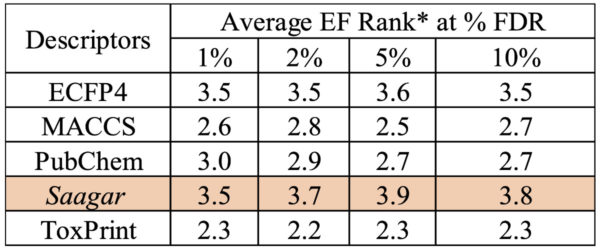
Interpretable QSPR Models with Saagar
Chemically aware Saagar substructures and moieties are interpretable by design, and are, thus, suited for interpretable QSPR models by providing chemistry-based reasoning for a prediction. Interpretability of QSAR models using Saagar features and Recursive Partitioning methodology is clearly demonstrated in the Tox21 vitamin D receptor (VDR) agonist assay model below. This model predicts whether a given chemical will be “active” (agonist) or “inactive” (not an agonist) of the VDR. Nodes with higher percentages of inactives are shown in blue, while nodes with higher percentages of actives are shown in green; darker shading reflects increased purity.
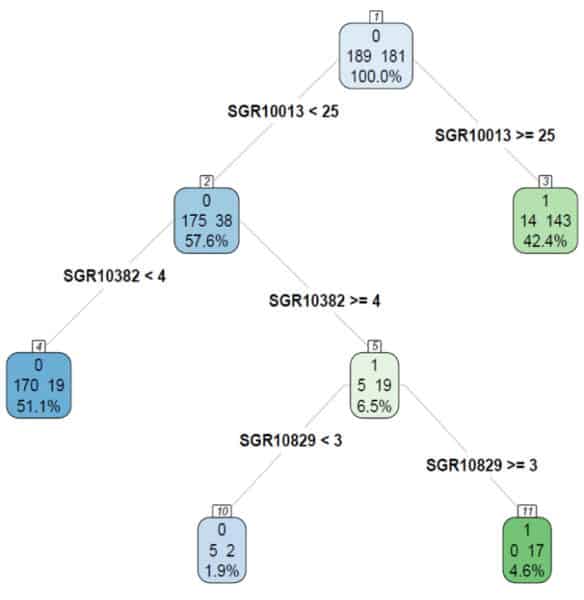
In this relatively simple, 3-layer deep model developed from 370 compounds, the core node (1) has almost 50-50 distribution of inactives and actives (189 inactives and 181 actives). The Saagar feature SGR10013, which represents the number of C atoms in a molecule, splits the core node into children nodes (2) and (3) with 213 (57.6%) and 157 (42.4%) compounds, respectively. Node 2 (SGR10013 <25) has mostly inactives (175/213 or 82.2% inactives), while node 3 (SGR10013 >=25) has mostly actives (143/157 or 91.1% actives). Thus, one can infer that larger molecules with 25 or more C atoms are likely to be VDR agonists. This structure-based reasoning would have correctly predicted Elocalcitol and Elgocalciferol as VDR agonists, though these two compounds were not part of the 370-compound training set in this example.
However, one must be careful about predictions from such simple, global rules. What if our query compound were a straight chain hydrocarbon with 30 C atoms? This model would falsely predict it to be a VDR agonist. Therefore, before accepting such a prediction, the overall “similarity” of the query molecule with the compounds in the node 3 must be determined as an applicability domain check.
Furthermore, individual predictions can be refined by considering multiple features. For example, in node 2, all compound with <25 C atoms are not inactive; in fact, 38 of the 213 compounds (~18%) are active. Two additional Saagar features, SGR10382 and SGR10829, can identify 17 of those 38 actives in node 11 with 100% purity. A representative compound, CHEMBL 116438, is shown, with SGR10382 highlighted in blue and SGR10829 highlighted in red.
Saagar-based RP models can also be used to help design compounds with desired properties. For example, if one were looking for compounds that are not agonists of VDR, the starting point could be a compound with <25 C atoms and fewer than 4 occurrences of the group with O connected to the middle C of a 3-C chain (SGR10382), because 90% (170/189) of such compounds are inactive in the VDR agonist assay (node 4).
Though the VDR model shown is relatively simple, it demonstrates how the recursive partitioning models built with Saagar features provide chemistry-based reasoning that can be used to predict biological activity or design compounds with desired properties, with confidence assigned based on prediction accuracies at each node. Notably, the Saagar set is extensible and because Saagar substructures and motifs are coded in SMARTS, they can be processed independent of any third-party software.
Publications and Presentations
Ford, L.C., Lin, H-C., Tsai, H-H. D., Zhou, Y-H., Wright, F.A., Sedykyh, A., Shah, R.R., Chiu, W.A. & Rusyn, I. (2024). Hazard and risk characterization of 56 structurally diverse PFAS using a targeted battery of broad coverage assays using six human cell types. Toxicology 503:153763.
Sedykh A.Y.; Shah R.R.; Kleinstreuer N.C.; Auerbach S.S.; Gombar V.K. (2020). Saagar – A New, Extensible Set of Molecular Substructures for QSAR/QSPR and Read-Across Predictions. Chemical Research in Toxicology.
V. Gombar1, R. Shah1, N. Kleinstreuer2, S. Auerbach2, A. Merrick2, A. Sedykh1 (2020). A new, extensible set of molecular substructures for interpretable QSARs and read-across applications. (Oral presentation at the American Chemical Society (ACS) Fall 2020 National Meeting & Expo [virtual].)
A. Sedykh1, R. Shah1, N. Kleinstreuer2, A. Merrick2, R. Paules2, S. Auerbach2, V. Gombar1 (2020). Interpretable QSAR and read-across predictions using Saagar – A new, extensible set of molecular substructures. (Accepted poster presentation in the QSAR2020 Conference, Durham, NC.)
V. Gombar1, R. Shah1, A. Merrick2, R. Paules2, S. Auerbach2, N. Kleinstreuer2, A. Sedykh1 (2020). Interpretable QSAR Models of Tox21 Assays (Accepted poster presentation at the 11th World Congress on Alternatives and Animal Use in the Life Sciences (WC11), Maastricht, The Netherlands.)
V. Gombar1, R. Shah1, A. Merrick2, R. Paules2, S. Auerbach2, N. Kleinstreuer2, and A. Sedykh1 (2020). Interpretable QSAR Models of Tox21 Assays. (Accepted poster presentation in the Society of Toxicology Meeting, Anaheim, CA.)
1 Sciome LLC, Research Triangle Park, NC, United States
2 National Institute of Environmental Health Sciences (NIEHS), National Toxicology Program (NTP), Research Triangle Park, NC, United States.