Molecular structure-based predictive models provide a proven alternative to costly and inefficient animal testing. However, due to a lack of interpretability of predictive models built with abstract molecular descriptors they have earned the notoriety of being black boxes. Interpretable models require interpretable descriptors to provide chemistry-backed predictive reasoning and facilitate intelligent molecular design. Sciome has developed a novel set of extensible chemistry-aware substructures, Saagar, to support interpretable predictive models and read-across protocols. In a recent collaborative study with NIEHS, now published in Chemical Research in Toxicology, “Saagar – A New, Extensible Set of Molecular Substructures for QSAR/QSPR and Read-Across Predictions,” Saagar features outperformed publicly available fingerprint/benchmark sets. Saagar features are interpretable and efficiently characterize diverse chemical collections, thus making them a better choice for building interpretable predictive in silico models and read-across protocols.
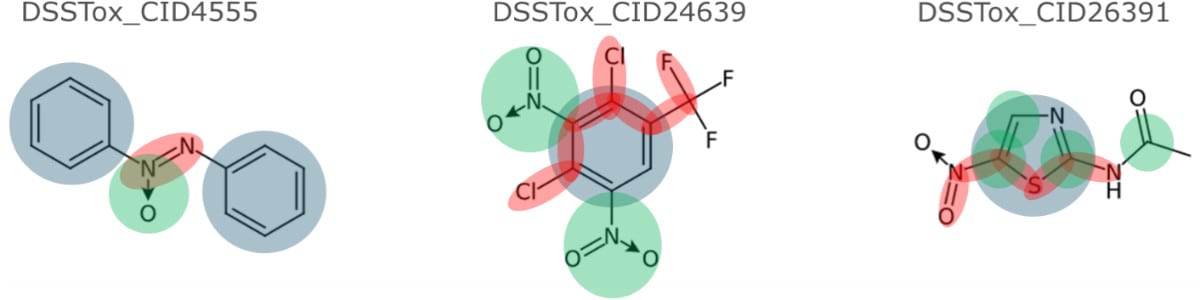